|

The combined, unsupervised analysis of coupled data sources is an open problem in machine learning. A particularly important example from the biological domain is the analysis of mRNA and protein profiles derived from the same set of genes (either over time or under different conditions). Such analysis has the potential to provide a far more comprehensive picture of the mechanisms of transcription and translation than the individual analysis of the separate data sets. The problem is similar to that attacked with traditional Canonical Correlation Analysis (CCA) but in many application areas, the CCA assumptions are too restrictive. Probabilistic CCA [1] and kernel CCA [2] have both been recently proposed but the former is still limited to linear relationships and the latter compromises the interpretability in the original space. In this work, we preset a nonparametric model for coupled data that provides an interpretable description of the shared variability in the data (as well as that that isn’t shared) whilst being free of restrictive assumptions such as those found in CCA. The hierarchical model is built from two marginal mixtures (one for each representation - generalisation to three or more is straightforward). Each object will be assigned to one component in each marginal and the contingency table describing these joint assignments is assumed to have been generated by a mixture of tables with independent margins. This top-level mixture captures the shared variability whilst the marginal models are free to capture variation specific to the respective data sources. The number of components in all three mixtures is inferred from the data using a novel Dirichlet Process (DP) formulation.
|
Video Length: 0
Date Found: October 12, 2010
Date Produced: December 20, 2008
|
|
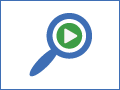 VideoLectures |
July 10, 2011
The explosion in growth of the Web of Linked Data has provided, for the first time, a plethora of information in disparate locations, yet bound together by machine-readable, semantically typed relations. Utilisation of the Web of Data has been, until now, restricted to members of the community, ...
|
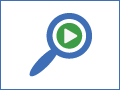 VideoLectures |
July 10, 2011
Problems cannot be solved with the mentality that has caused them’. Hence, the 2008- crisis cannot be solved with ethics of one-sided and short-term mentality of the industrial and neoliberal economics, which has caused the ‘Bubble Economy’ of several recent decades. Neither the market nor the ...
|
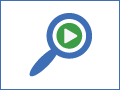 VideoLectures |
July 10, 2011
|
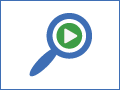 VideoLectures |
July 10, 2011
|
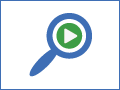 VideoLectures |
July 10, 2011
Social media presents unique challenges for topic classification, including the brevity of posts, the informal nature of conversations, and the frequent reliance on external hyperlinks to give context to a conversation. In this paper we investigate the usefulness of these external hyperlinks ...
|
|
|
|
|
|
Featured Content
Featuring websites that enhance the internet user’s experience.
|